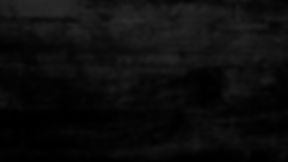
USING ARTIFICIAL INTELLIGENCE TO COMBAT FAKE NEWS
Research Abstract
A New Conceptual Framework for the Analysis of Fake News Using Artificial Intelligence
The spread of Fake News in the 2016 presidential election presented a major threat to the integrity of American democracy. Computational social scientists and journalists alike have rushed to define the scope of the Fake News phenomenon. However, due to a lack of empirical data and a dearth of a standardized vocabulary, current Fake News definitions are rife with subjectivity and vary between research, making major papers incomparable. The spread of internationally based disinformation has been documented as a continued threat in the upcoming election cycle, yet the failure of the scientific community to define Fake News has hindered policy proposals. I hypothesized that a multi-faceted classification for Fake News would perform better than conventional binary definitions. To test this, a new conceptual Fake News framework consisting of a 4 step classifier was devised. I applied this new definition to a sample of a dataset of 12.8k labeled Fake News headlines, and used the logit regression machine learning model to predict fake articles and measure the efficacy of the classifier. Using a confusion matrix, my results outperformed previous models on both precision and recall, producing a 45% jump in precision from existing research on the same dataset. This research could be used to produce a generalized Fake News predictive algorithm, which could help arm the public with the tools to identify misinformation on the internet.